MACS lab attended IROS 2024 conference
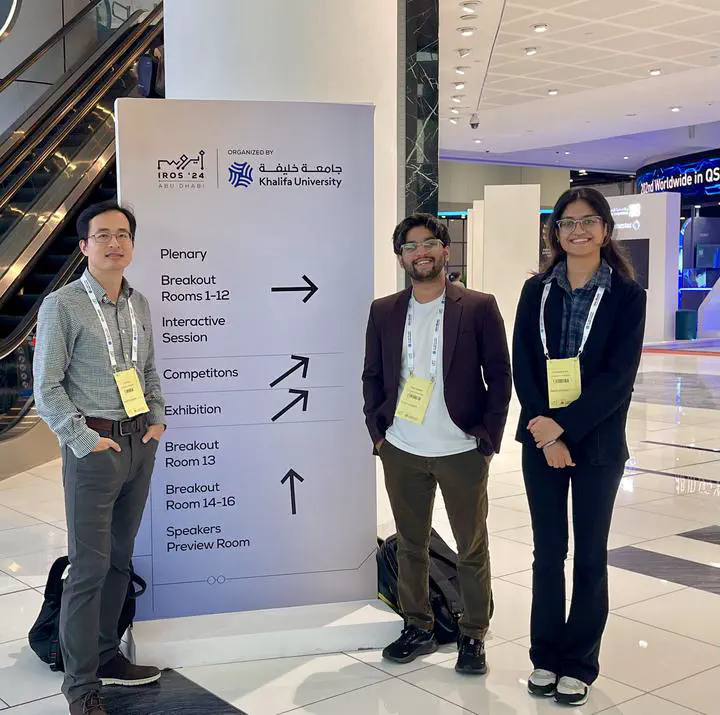
Excited that our slip detection and robotic grasping work were presented as a regular paper there. Safely handling objects and avoiding slippage are fundamental challenges in robotic manipulation, yet traditional techniques often oversimplify the issue by treating slippage as a binary occurrence. Our research presents a framework that both identifies slip incidents and measures their severity. We introduce a set of features based on detailed vector field analysis of tactile deformation data captured by the GelSight Mini sensor. Two distinct machine learning models use these features: one focuses on slip detection, and the other on evaluating the slip’s severity which is the slipping velocity of the object against the sensor surface. Our slip detection model achieves an average accuracy of 92%, and the slip severity estimation model exhibits a mean absolute error (MAE) of 0.6 cm per second for unseen objects. To demonstrate the synergistic approach of this framework, we employ both of these models in tactile feedback-guided vertical sliding tasks. Leveraging the high accuracy of slip detection, we utilize it as the foundational and corrective model and integrate the slip severity score into the feedback control loop to address slips without overcompensating.
More at https://sites.google.com/uw.edu/lsds.